Do you start feeling all yucky and drab come fall time because all of your plants will start turning dead and icky colors?
As the weather starts colder, our once gorgeous plants will start turning all yellowish-brown, and the blooms will start falling off, but are we supposed to leave them there?
No, once the plants turn yellowish-brown and slowly start showing, you need to prune them back or pull them off.
Why do you ask? If you leave the dead on your plants, not only will it look bad, but also your plant will continue to send nutrients to that vein or stem, which means if that part is already dead, your plant is wasting nutrients when it could be giving those good nutrients to the still living part.
When we prune our plants, trees, and shrubs, it makes them look better and makes them grow healthier. Pruning can be done with a simple pair of pruning shears or scissors. You would be amazed at how a few minutes can go a long way.
Source of Information on Pruning
https://www.tnnursery.net
Pruning: Trimming the Excess to Reveal Information
In data science and machine learning, where abundant information often obscures the signal amidst the noise, pruning emerges as a crucial technique. It's a process akin to gardening, where selective removal of branches allows the plant to flourish. Similarly, in machine learning models, pruning involves selectively removing unnecessary parameters or structures to enhance efficiency, reduce complexity, and reveal the essential information embedded within the data. This article delves into the depths of pruning, exploring its various forms, applications, and significance in uncovering information.
Understanding Pruning
Pruning is a crucial technique used in machine learning to optimize and refine models by eliminating redundant or irrelevant components. It operates on the idea that not all parameters or connections are equally crucial for model performance. By identifying and removing such expendable elements, pruning aims to improve efficiency without compromising accuracy.
To understand how pruning works, we must know that machine learning models are complex structures with many components. These components can be parameters, connections, or even complete layers. These components are adjusted during training to maximize the model's performance on a given task. However, not all of them are equally important, and some may even hinder the model's performance by introducing noise or overfitting.
Pruning addresses this issue by removing the least essential components. There are different ways to determine the importance of an element, but the most common method is to measure its contribution to the model's performance. For instance, a parameter that has little impact on the model's accuracy can be removed without affecting its performance significantly.
Pruning can be performed at different stages of the model's lifecycle, from the initial design to the post-training phase.
Types of Pruning
Weight Pruning: This technique involves identifying and eliminating connections in neural networks with negligible weights. By setting these weights to zero or near-zero values, weight pruning reduces the model's parameter count, thus minimizing computational overhead.
Unit Pruning: Unit pruning targets entire neurons or units within a neural network that contribute minimally to the model's output. Removing these redundant units decreases the network's size, leading to faster inference and reduced memory requirements.
Filter Pruning: In convolutional neural networks (CNNs), filter pruning involves removing entire convolutional filters that contribute insignificantly to feature extraction. Filter pruning reduces the model's computational cost while preserving its representative capacity by eliminating redundant filters.
Structured Pruning: Unlike random pruning, structured pruning removes entire structured components, such as channels, layers, or blocks, from a neural network. This method maintains the model's structural integrity while reducing its size and computational complexity.
Techniques for Pruning
Magnitude-based Pruning: This approach involves ranking parameters based on their magnitudes and removing those with the smallest values. Magnitude-based pruning is simple yet effective, as it targets parameters least likely to influence the model's output significantly.
L1/L2 Regularization: Regularization techniques, such as L1 and L2 regularization, penalize large parameter values during training. By imposing sparsity constraints on the model's weights, regularization encourages the emergence of sparse representations, facilitating subsequent pruning.
Fine-tuning: After pruning redundant parameters, fine-tuning involves retraining the pruned model on the original dataset to restore its performance. Fine-tuning allows the model to compensate for the removed parameters and adapt to the revised network structure.
Applications of Pruning
Pruning finds applications across various domains and disciplines, owing to its ability to enhance model efficiency and interpretability while maintaining or improving performance.
Resource-Constrained Environments
Computational resources, such as mobile devices or embedded systems, are limited in resource-constrained environments. Pruning enables the deployment of efficient models tailored to such constraints, ensuring optimal performance without exceeding resource constraints.
Edge Computing
Edge computing environments, characterized by their decentralized nature and limited computational resources, benefit from lightweight models enabled by pruning. Pruning facilitates inference at the network edge by reducing model size and complexity, minimizing latency, and conserving bandwidth.
Interpretability and Explainability
Pruned models often exhibit increased interpretability, as removing redundant parameters simplifies the model's structure and decision-making process. Interpretability is particularly crucial in domains such as healthcare and finance, where model transparency and explainability are paramount.
Federated Learning Pruning
In federated learning settings, where models are trained across distributed devices or servers, pruning enhances communication efficiency by reducing the size of model updates transmitted between nodes. Pruned models require fewer parameters to be exchanged, mitigating communication overhead and accelerating convergence.
Challenges and Considerations
While pruning offers substantial benefits regarding model efficiency and interpretability, it has challenges and considerations.
Loss of Information
Aggressive pruning may result in the loss of valuable information encoded within the discarded parameters. Balancing the trade-off between model compression and information retention is critical to ensure that pruning does not compromise performance or predictive accuracy.
Sensitivity to Initialization
Pruning techniques often rely on initialization schemes and hyperparameters that may influence the effectiveness of the pruning process. Sensitivity to initialization requires careful tuning to optimize pruning outcomes and mitigate the risk of performance degradation.
Non-Uniform Impact (Layering Pruning)
Pruning may have a non-uniform impact on different layers or components of a neural network. Specific layers or units may be more critical to the model's performance, necessitating adaptive pruning strategies that account for such essential variations.
Relevance to Sparse Data
The efficacy of pruning techniques may vary depending on the sparsity and distribution of the input data. Sparse data may pose challenges for pruning algorithms, as the relevance and significance of parameters may be less discernible in light regions of the input space.
Pruning is a powerful tool in the machine learning practitioner's arsenal, offering a means to distill complex models into leaner, more efficient counterparts. By selectively removing redundant parameters and structures, pruning reveals the essential information embedded within the data, facilitating faster inference, reduced resource consumption, and enhanced interpretability. As machine learning continues to permeate various domains and applications, the importance of pruning in optimizing model performance and efficiency remains unequivocal. Through ongoing research and innovation, pruning techniques will continue to evolve, enabling the development of increasingly efficient and interpretable machine learning models.
Read more
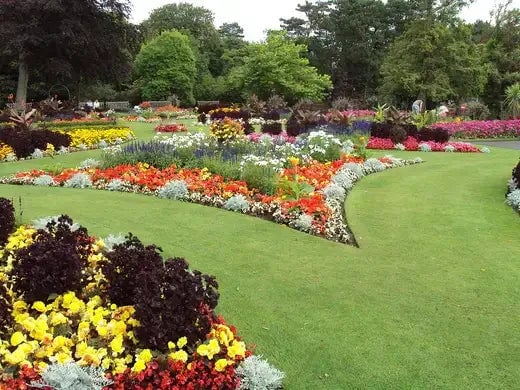
Living Art- How to Choose Colors in a Garden Choosing colors for a garden should be fun, not work. However, with so many choices, a person can become easily confused. Coordinating flowers is about ...
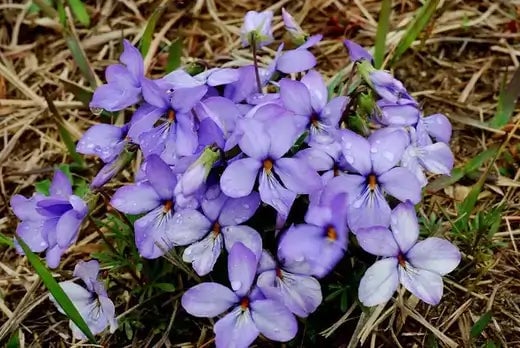
The Viola Pedata, or as it is also called Birds foot violet, is a perennial mountain plant that grows in northeastern American areas.The Appalachian mountain range is the leading home for these wil...